Hyper-optimized tensor network contraction
1Blackett Laboratory, Imperial College London, London SW7 2AZ, United Kingdom
2Division of Chemistry and Chemical Engineering, California Institute of Technology, Pasadena, California 91125, USA
3Department of Physics, Boston University, Boston, MA, 02215, USA
4Institut quantique & Département de physique, Université de Sherbrooke, Québec J1K 2R1, Canada
Published: | 2021-03-15, volume 5, page 410 |
Eprint: | arXiv:2002.01935v4 |
Doi: | https://doi.org/10.22331/q-2021-03-15-410 |
Citation: | Quantum 5, 410 (2021). |
Find this paper interesting or want to discuss? Scite or leave a comment on SciRate.
Abstract
Tensor networks represent the state-of-the-art in computational methods across many disciplines, including the classical simulation of quantum many-body systems and quantum circuits. Several applications of current interest give rise to tensor networks with irregular geometries. Finding the best possible contraction path for such networks is a central problem, with an exponential effect on computation time and memory footprint. In this work, we implement new randomized protocols that find very high quality contraction paths for arbitrary and large tensor networks. We test our methods on a variety of benchmarks, including the random quantum circuit instances recently implemented on Google quantum chips. We find that the paths obtained can be very close to optimal, and often many orders or magnitude better than the most established approaches. As different underlying geometries suit different methods, we also introduce a hyper-optimization approach, where both the method applied and its algorithmic parameters are tuned during the path finding. The increase in quality of contraction schemes found has significant practical implications for the simulation of quantum many-body systems and particularly for the benchmarking of new quantum chips. Concretely, we estimate a speed-up of over 10,000$\times$ compared to the original expectation for the classical simulation of the Sycamore `supremacy' circuits.
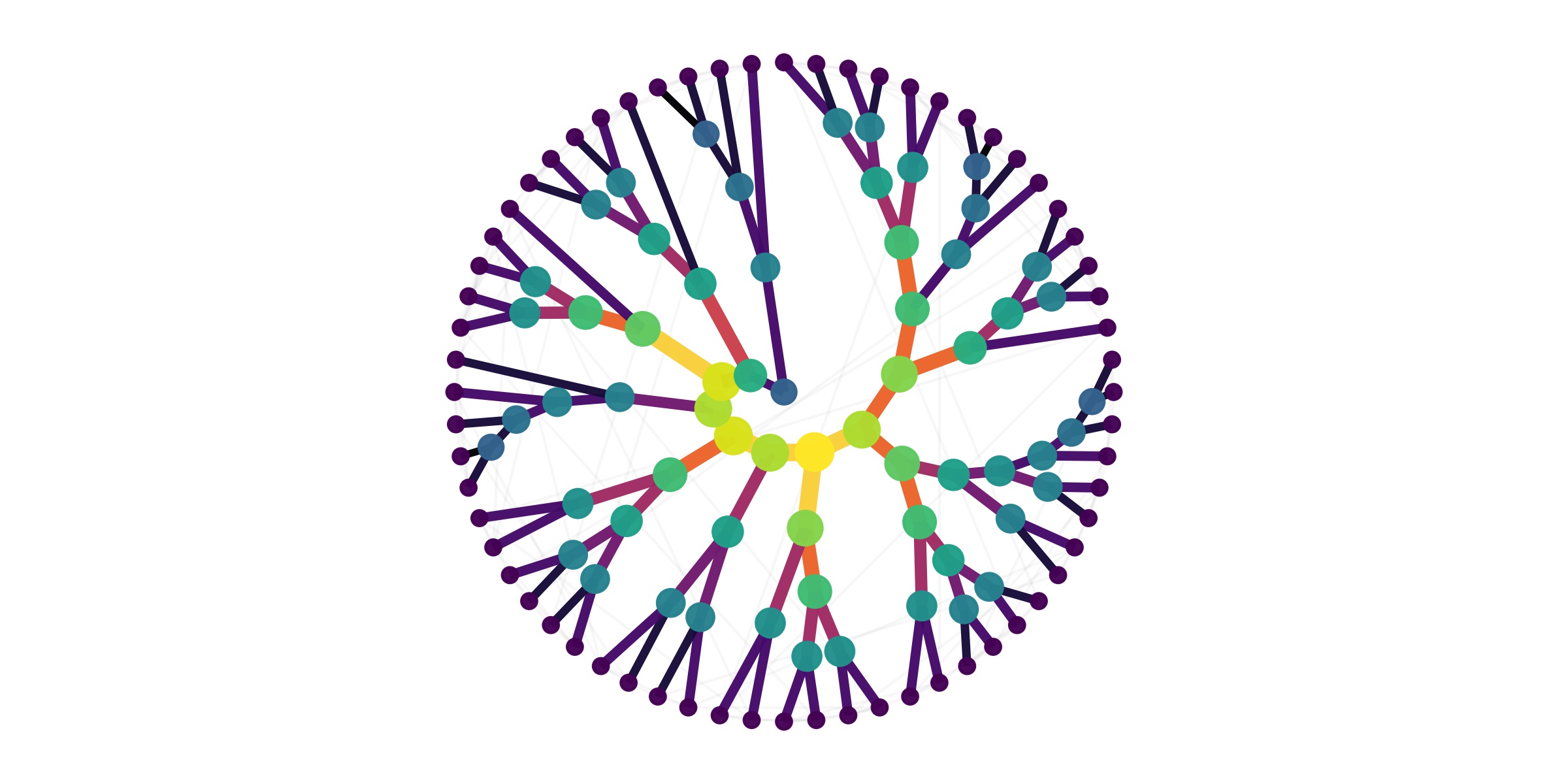
Featured image: Example of a near-optimal contraction tree for a small tensor network.
Popular summary
► BibTeX data
► References
[1] F. Verstraete, V. Murg, and J. Cirac, Adv. Phys. 57, 143 (2008).
https://doi.org/10.1080/14789940801912366
[2] R. Orús, Ann. Phys. (N. Y). 349, 117 (2014).
https://doi.org/10.1016/j.aop.2014.06.013
[3] J. C. Bridgeman and C. T. Chubb, J. Phys. A Math. Theor. 50, 223001 (2017).
https://doi.org/10.1088/1751-8121/aa6dc3
[4] J. D. Biamonte and V. Bergholm, arXiv:1708.00006 (2017), arXiv:1708.00006.
arXiv:1708.00006
[5] M. Levin and C. P. Nave, Phys. Rev. Lett. 99, 120601 (2007).
https://doi.org/10.1103/PhysRevLett.99.120601
[6] G. Evenbly and G. Vidal, Phys. Rev. Lett. 115, 180405 (2015).
https://doi.org/10.1103/PhysRevLett.115.180405
[7] G. Evenbly, Phys. Rev. B 95, 045117 (2017).
https://doi.org/10.1103/PhysRevB.95.045117
[8] A. Cichocki, N. Lee, I. Oseledets, A.-H. Phan, Q. Zhao, and D. P. Mandic, Found. Trends Mach. Learn. 9, 249 (2016).
https://doi.org/10.1561/2200000059
[9] A. Cichocki, N. Lee, I. Oseledets, A.-H. Phan, Q. Zhao, M. Sugiyama, and D. P. Mandic, Found. Trends Mach. Learn. 9, 431 (2017).
https://doi.org/10.1561/2200000067
[10] L. Dueñas-Osorio, M. Y. Vardi, and J. Rojo, Struct. Saf. 75, 110 (2018).
https://doi.org/10.1016/j.strusafe.2018.05.004
[11] I. L. Markov and Y. Shi, SIAM J. Comput. 38, 963 (2008).
https://doi.org/10.1137/050644756
[12] E. Stoudenmire and D. J. Schwab, in Advances in Neural Information Processing Systems 29, edited by D. D. Lee, M. Sugiyama, U. V. Luxburg, I. Guyon, and R. Garnett (Curran Associates, Inc., 2016) pp. 4799–4807.
http://papers.nips.cc/paper/6211-supervised-learning-with-tensor-networks.pdf
[13] E. M. Stoudenmire, Quantum Sci. Technol. 3, 034003 (2018).
https://doi.org/10.1088/2058-9565/aaba1a
[14] C. Roberts, A. Milsted, M. Ganahl, A. Zalcman, B. Fontaine, Y. Zou, J. Hidary, G. Vidal, and S. Leichenauer, arXiv:1905.01330 (2019), arXiv:1905.01330.
arXiv:1905.01330
[15] H. C. Jiang, Z. Y. Weng, and T. Xiang, Phys. Rev. Lett. 101, 090603 (2008).
https://doi.org/10.1103/PhysRevLett.101.090603
[16] Z.-C. Gu and X.-G. Wen, Phys. Rev. B 80, 155131 (2009).
https://doi.org/10.1103/PhysRevB.80.155131
[17] Z. Y. Xie, J. Chen, M. P. Qin, J. W. Zhu, L. P. Yang, and T. Xiang, Phys. Rev. B 86, 045139 (2012).
https://doi.org/10.1103/PhysRevB.86.045139
[18] H.-H. Zhao, Z. Y. Xie, T. Xiang, and M. Imada, Phys. Rev. B 93, 125115 (2016).
https://doi.org/10.1103/PhysRevB.93.125115
[19] M. Bal, M. Mariën, J. Haegeman, and F. Verstraete, Phys. Rev. Lett. 118, 250602 (2017).
https://doi.org/10.1103/PhysRevLett.118.250602
[20] S. Yang, Z.-C. Gu, and X.-G. Wen, Phys. Rev. Lett. 118, 110504 (2017).
https://doi.org/10.1103/PhysRevLett.118.110504
[21] Y.-Y. Shi, L.-M. Duan, and G. Vidal, Phys. Rev. A 74, 022320 (2006).
https://doi.org/10.1103/PhysRevA.74.022320
[22] L. G. Valiant, SIAM J. Comput. 37, 1565 (2008).
https://doi.org/10.1137/070682575
[23] S. Bravyi, Contemp. Math. 482, 179 (2008), arXiv:0801.2989.
https://doi.org/10.1090/conm/482/09419
arXiv:0801.2989
[24] M. Aguado and G. Vidal, Phys. Rev. Lett. 100, 070404 (2008).
https://doi.org/10.1103/PhysRevLett.100.070404
[25] R. König, B. W. Reichardt, and G. Vidal, Phys. Rev. B 79, 195123 (2009).
https://doi.org/10.1103/PhysRevB.79.195123
[26] S. J. Denny, J. D. Biamonte, D. Jaksch, and S. R. Clark, J. Phys. A Math. Theor. 45, 015309 (2012).
https://doi.org/10.1088/1751-8113/45/1/015309
[27] L. G. Valiant, Theor. Comput. Sci. 8, 189 (1979).
https://doi.org/10.1016/0304-3975(79)90044-6
[28] C. Damm, M. Holzer, and P. McKenzie, Comput. Complex. 11, 54 (2002).
https://doi.org/10.1007/s00037-000-0170-4
[29] B. M. Terhal and D. P. DiVincenzo, Quant. Inf. Comp. 4, 134 (2004), arXiv:0205133 [quant-ph].
arXiv:quant-ph/0205133
[30] M. J. Bremner, R. Jozsa, and D. J. Shepherd, Proc. R. Soc. A Math. Phys. Eng. Sci. 467, 459 (2010).
https://doi.org/10.1098/rspa.2010.0301
[31] S. Aaronson and A. Arkhipov, Theory Comput. 9, 143 (2013).
https://doi.org/10.4086/toc.2013.v009a004
[32] R. Jozsa and M. V. den Nest, arXiv:1305.6190 (2013), https://doi.org/10.26421/qic14.7-8-7, arXiv:1305.6190.
https://doi.org/10.26421/qic14.7-8-7
arXiv:1305.6190
[33] T. Morimae, K. Fujii, and J. F. Fitzsimons, Phys. Rev. Lett. 112, 130502 (2014).
https://doi.org/10.1103/PhysRevLett.112.130502
[34] J. Carolan, C. Harrold, C. Sparrow, E. Martin-Lopez, N. J. Russell, J. W. Silverstone, P. J. Shadbolt, N. Matsuda, M. Oguma, M. Itoh, G. D. Marshall, M. G. Thompson, J. C. F. Matthews, T. Hashimoto, J. L. O'Brien, and A. Laing, Science 349, 711 (2015).
https://doi.org/10.1126/science.aab3642
[35] E. Farhi and A. W. Harrow, arXiv:1602.07674 (2016), arXiv:1602.07674.
arXiv:1602.07674
[36] S. Aaronson, A. Bouland, G. Kuperberg, and S. Mehraban, in Proceedings of the 49th Annual ACM SIGACT Symposium on Theory of Computing (2017) pp. 317–327.
https://doi.org/10.1145/3055399.3055453
[37] S. Aaronson and L. Chen, arXiv:1612.05903 (2016), arXiv:1612.05903.
arXiv:1612.05903
[38] S. Boixo, S. V. Isakov, V. N. Smelyanskiy, R. Babbush, N. Ding, Z. Jiang, M. J. Bremner, J. M. Martinis, and H. Neven, Nat. Phys. 14, 595 (2018).
https://doi.org/10.1038/s41567-018-0124-x
[39] A. Bouland, B. Fefferman, C. Nirkhe, and U. Vazirani, Nat. Phys. 15, 159 (2019).
https://doi.org/10.1038/s41567-018-0318-2
[40] E. S. Fried, N. P. D. Sawaya, Y. Cao, I. D. Kivlichan, J. Romero, and A. Aspuru-Guzik, PLoS One 13, e0208510 (2018).
https://doi.org/10.1371/journal.pone.0208510
[41] J. Chen, F. Zhang, C. Huang, M. Newman, and Y. Shi, arXiv:1805.01450 (2018), arXiv:1805.01450.
arXiv:1805.01450
[42] E. F. Dumitrescu, A. L. Fisher, T. D. Goodrich, T. S. Humble, B. D. Sullivan, and A. L. Wright, PLoS One 13, e0207827 (2018).
https://doi.org/10.1371/journal.pone.0207827
[43] S. Kourtis, C. Chamon, E. R. Mucciolo, and A. E. Ruckenstein, SciPost Phys. 7, 60 (2019).
https://doi.org/10.21468/SciPostPhys.7.5.060
[44] J. M. Dudek, L. Dueñas-Osorio, and M. Y. Vardi, arXiv:1908.04381 (2019), arXiv:1908.04381.
arXiv:1908.04381
[45] F. Arute, K. Arya, R. Babbush, D. Bacon, J. C. Bardin, R. Barends, R. Biswas, S. Boixo, F. G. S. L. Brandao, D. A. Buell, B. Burkett, Y. Chen, Z. Chen, B. Chiaro, R. Collins, W. Courtney, A. Dunsworth, E. Farhi, B. Foxen, A. Fowler, C. Gidney, M. Giustina, R. Graff, K. Guerin, S. Habegger, M. P. Harrigan, M. J. Hartmann, A. Ho, M. Hoffmann, T. Huang, T. S. Humble, S. V. Isakov, E. Jeffrey, Z. Jiang, D. Kafri, K. Kechedzhi, J. Kelly, P. V. Klimov, S. Knysh, A. Korotkov, F. Kostritsa, D. Landhuis, M. Lindmark, E. Lucero, D. Lyakh, S. Mandrà, J. R. McClean, M. McEwen, A. Megrant, X. Mi, K. Michielsen, M. Mohseni, J. Mutus, O. Naaman, M. Neeley, C. Neill, M. Y. Niu, E. Ostby, A. Petukhov, J. C. Platt, C. Quintana, E. G. Rieffel, P. Roushan, N. C. Rubin, D. Sank, K. J. Satzinger, V. Smelyanskiy, K. J. Sung, M. D. Trevithick, A. Vainsencher, B. Villalonga, T. White, Z. J. Yao, P. Yeh, A. Zalcman, H. Neven, and J. M. Martinis, Nature 574, 505 (2019).
https://doi.org/10.1038/s41586-019-1666-5
[46] D. Bienstock, Journal of Combinatorial Theory, Series B 49, 103 (1990).
https://doi.org/10.1016/0095-8956(90)90066-9
[47] B. O'Gorman, in 14th Conference on the Theory of Quantum Computation, Communication and Cryptography (TQC 2019), Leibniz International Proceedings in Informatics (LIPIcs), Vol. 135, edited by W. van Dam and L. Mancinska (Schloss Dagstuhl–Leibniz-Zentrum fuer Informatik, Dagstuhl, Germany, 2019) pp. 10:1–10:19.
https://doi.org/10.4230/LIPIcs.TQC.2019.10
[48] R. N. C. Pfeifer, J. Haegeman, and F. Verstraete, Phys. Rev. E 90, 033315 (2014).
https://doi.org/10.1103/PhysRevE.90.033315
[49] D. G. A. Smith and J. Gray, J. Open Source Softw. 3, 753 (2018).
https://doi.org/10.21105/joss.00753
[50] V. Gogate and R. Dechter, in Proceedings of the 20th conference on Uncertainty in artificial intelligence (AUAI Press, 2004) pp. 201–208, arXiv:1207.4109.
arXiv:1207.4109
[51] M. Hamann and B. Strasser, J. Exp. Algorithmics 23, 1.2 (2018).
https://doi.org/10.1145/3173045
[52] B. Strasser, arXiv:1709.08949 (2017), arXiv:1709.08949.
arXiv:1709.08949
[53] M. A. Porter, J.-P. Onnela, and P. J. Mucha, Not. Am. Math. Soc. 56, 1082 (2009), arXiv:0902.3788.
arXiv:0902.3788
[54] S. Fortunato, Phys. Rep. 486, 75 (2010).
https://doi.org/10.1016/j.physrep.2009.11.002
[55] M. Girvan and M. E. J. Newman, Proc. Natl. Acad. Sci. 99, 7821 (2002).
https://doi.org/10.1073/pnas.122653799
[56] S. Schlag, V. Henne, T. Heuer, H. Meyerhenke, P. Sanders, and C. Schulz, in 2016 Proc. Eighteenth Work. Algorithm Eng. Exp. (Society for Industrial and Applied Mathematics, Philadelphia, PA, 2016) pp. 53–67.
https://doi.org/10.1137/1.9781611974317.5
[57] Y. Akhremtsev, T. Heuer, P. Sanders, and S. Schlag, in 2017 Proc. Ninteenth Work. Algorithm Eng. Exp. (Society for Industrial and Applied Mathematics, Philadelphia, PA, 2017) pp. 28–42.
https://doi.org/10.1137/1.9781611974768.3
[58] D. A. Papa and I. L. Markov, in Handbook of Approximation Algorithms and Metaheuristics (2007).
[59] B. Shahriari, K. Swersky, Z. Wang, R. P. Adams, and N. de Freitas, Proceedings of the IEEE 104, 148 (2016).
https://doi.org/10.1109/JPROC.2015.2494218
[60] L. Gustafson, Bayesian Tuning and Bandits: An Extensible, Open Source Library for AutoML, M. eng thesis, Massachusetts Institute of Technology, Cambridge, MA (2018).
https://dai.lids.mit.edu/wp-content/uploads/2018/05/Laura_MEng_Final.pdf
[61] C. K. Williams and C. E. Rasmussen, Gaussian processes for machine learning, Vol. 2 (MIT press Cambridge, MA, 2006).
https://doi.org/10.7551/mitpress/3206.001.0001
[62] S. Boixo, S. V. Isakov, V. N. Smelyanskiy, and H. Neven, arXiv:1712.05384 (2017), arXiv:1712.05384.
arXiv:1712.05384
[63] J. Gray, Journal of Open Source Software 3, 819 (2018).
https://doi.org/10.21105/joss.00819
[64] J. Gray, ``cotengra,'' https://github.com/jcmgray/cotengra (2020).
https://github.com/jcmgray/cotengra
[65] B. Villalonga, D. Lyakh, S. Boixo, H. Neven, T. S. Humble, R. Biswas, E. G. Rieffel, A. Ho, and S. Mandrà, Quantum Science and Technology 5, 034003 (2020).
https://doi.org/10.1088/2058-9565/ab7eeb
[66] I. L. Markov and Y. Shi, Algorithmica 59 (2009), https://doi.org/10.1007/s00453-009-9312-5.
https://doi.org/10.1007/s00453-009-9312-5
[67] F. Viger and M. Latapy, in COCOON'05 Proc. 11th Annu. Int. Conf. Comput. Comb. (2005) pp. 440–449.
https://doi.org/10.1007/11533719_45
[68] K. Meichanetzidis and S. Kourtis, Phys. Rev. E 100, 033303 (2019).
https://doi.org/10.1103/PhysRevE.100.033303
[69] É. Fusy, Random Struct. Algorithms 35, 464 (2009).
https://doi.org/10.1002/rsa.20275
[70] J.-Y. Cai and V. Choudhary, Theoretical Computer Science 384, 22 (2007), theory and Applications of Models of Computation.
https://doi.org/10.1016/j.tcs.2007.05.015
[71] F. Bacchus, S. Dalmao, and T. Pitassi, in 44th Annual IEEE Symposium on Foundations of Computer Science, 2003. Proceedings. (2003) pp. 340–351.
https://doi.org/10.1109/SFCS.2003.1238208
[72] C. Domshlak and J. Hoffmann, J. Artif. Int. Res. 30, 565–620 (2007).
https://doi.org/10.1613/jair.2289
[73] C. P. Gomes, A. Sabharwal, and B. Selman, in Handbook of Satisfiability (2009).
[74] ``1st international competition on model counting (mc 2020),''.
https://mccompetition.org/2020/mc_description
[75] J. Dudek, V. Phan, and M. Vardi, ``Addmc: Weighted model counting with algebraic decision diagrams,'' (2020).
https://doi.org/10.1609/aaai.v34i02.5505
[76] E. Farhi, J. Goldstone, and S. Gutmann, (2014), arXiv:1411.4028 [quant-ph].
arXiv:1411.4028
[77] C. Huang, M. Szegedy, F. Zhang, X. Gao, J. Chen, and Y. Shi, (2019), arXiv:1909.02559 [quant-ph].
arXiv:1909.02559
[78] J. Preskill, arXiv:1203.5813 (2012), arXiv:1203.5813.
arXiv:1203.5813
[79] E. Pednault, J. A. Gunnels, G. Nannicini, L. Horesh, T. Magerlein, E. Solomonik, E. W. Draeger, E. T. Holland, and R. Wisnieff, arXiv:1710.05867 (2017), arXiv:1710.05867.
arXiv:1710.05867
[80] F. Zhang, C. Huang, M. Newman, J. Cai, H. Yu, Z. Tian, B. Yuan, H. Xu, J. Wu, X. Gao, J. Chen, M. Szegedy, and Y. Shi, arXiv:1907.11217 (2019), arXiv:1907.11217.
arXiv:1907.11217
[81] B. Villalonga, S. Boixo, B. Nelson, C. Henze, E. Rieffel, R. Biswas, and S. Mandrà, npj Quantum Inf. 5, 86 (2019).
https://doi.org/10.1038/s41534-019-0196-1
[82] C. Guo, Y. Liu, M. Xiong, S. Xue, X. Fu, A. Huang, X. Qiang, P. Xu, J. Liu, S. Zheng, H.-L. Huang, M. Deng, D. Poletti, W.-S. Bao, and J. Wu, Phys. Rev. Lett. 123, 190501 (2019).
https://doi.org/10.1103/PhysRevLett.123.190501
[83] I. L. Markov, A. Fatima, S. V. Isakov, and S. Boixo, arXiv preprint arXiv:1807.10749 (2018).
arXiv:1807.10749
[84] https://github.com/sboixo/GRCS.
https://github.com/sboixo/GRCS
[85] I. D. Kivlichan, J. McClean, N. Wiebe, C. Gidney, A. Aspuru-Guzik, G. K.-L. Chan, and R. Babbush, Phys. Rev. Lett. 120, 110501 (2018).
https://doi.org/10.1103/PhysRevLett.120.110501
[86] J. Bradbury, R. Frostig, P. Hawkins, M. J. Johnson, C. Leary, D. Maclaurin, and S. Wanderman-Milne, ``JAX: composable transformations of Python+NumPy programs,'' (2018).
http://github.com/google/jax
[87] S. Bravyi, M. Suchara, and A. Vargo, Phys. Rev. A 90, 032326 (2014).
https://doi.org/10.1103/PhysRevA.90.032326
[88] A. J. Ferris and D. Poulin, Phys. Rev. Lett. 113, 030501 (2014).
https://doi.org/10.1103/PhysRevLett.113.030501
[89] C. T. Chubb and S. T. Flammia, arXiv:1809.10704 (2018).
arXiv:1809.10704
Cited by
[1] Philipp Seitz, Ismael Medina, Esther Cruz, Qunsheng Huang, and Christian B. Mendl, "Simulating quantum circuits using tree tensor networks", Quantum 7, 964 (2023).
[2] Jin-Guo Liu, Xun Gao, Madelyn Cain, Mikhail D. Lukin, and Sheng-Tao Wang, "Computing Solution Space Properties of Combinatorial Optimization Problems Via Generic Tensor Networks", SIAM Journal on Scientific Computing 45 3, A1239 (2023).
[3] Salvatore Mandra, Jeffrey Marshall, Eleanor G. Rieffel, and Rupak Biswas, 2021 IEEE/ACM Second International Workshop on Quantum Computing Software (QCS) 99 (2021) ISBN:978-1-7281-8674-0.
[4] Feng Pan, Keyang Chen, and Pan Zhang, "Solving the Sampling Problem of the Sycamore Quantum Circuits", Physical Review Letters 129 9, 090502 (2022).
[5] Shi-Ju Ran and Gang Su, "Tensor Networks for Interpretable and Efficient Quantum-Inspired Machine Learning", Intelligent Computing 2, 0061 (2023).
[6] Harun Bayraktar, Ali Charara, David Clark, Saul Cohen, Timothy Costa, Yao-Lung L. Fang, Yang Gao, Jack Guan, John Gunnels, Azzam Haidar, Andreas Hehn, Markus Hohnerbach, Matthew Jones, Tom Lubowe, Dmitry Lyakh, Shinya Morino, Paul Springer, Sam Stanwyck, Igor Terentyev, Satya Varadhan, Jonathan Wong, and Takuma Yamaguchi, 2023 IEEE International Conference on Quantum Computing and Engineering (QCE) 1050 (2023) ISBN:979-8-3503-4323-6.
[7] Sergey Bravyi, David Gosset, and Yinchen Liu, "How to Simulate Quantum Measurement without Computing Marginals", Physical Review Letters 128 22, 220503 (2022).
[8] Lukas Burgholzer, Alexander Ploier, and Robert Wille, "Simulation Paths for Quantum Circuit Simulation With Decision Diagrams What to Learn From Tensor Networks, and What Not", IEEE Transactions on Computer-Aided Design of Integrated Circuits and Systems 42 4, 1113 (2023).
[9] Daniel Strano, Benn Bollay, Aryan Blaauw, Nathan Shammah, William J. Zeng, and Andrea Mari, 2023 IEEE International Conference on Quantum Computing and Engineering (QCE) 949 (2023) ISBN:979-8-3503-4323-6.
[10] Tuomas Laakkonen, Konstantinos Meichanetzidis, and John van de Wetering, "Picturing Counting Reductions with the ZH-Calculus", Electronic Proceedings in Theoretical Computer Science 384, 89 (2023).
[11] Thorsten B. Wahl and Sergii Strelchuk, "Simulating Quantum Circuits Using Efficient Tensor Network Contraction Algorithms with Subexponential Upper Bound", Physical Review Letters 131 18, 180601 (2023).
[12] Ar A Melnikov, A A Termanova, S V Dolgov, F Neukart, and M R Perelshtein, "Quantum state preparation using tensor networks", Quantum Science and Technology 8 3, 035027 (2023).
[13] Ramis Movassagh, "The hardness of random quantum circuits", Nature Physics 19 11, 1719 (2023).
[14] Willow Ahrens, Fredrik Kjolstad, and Saman Amarasinghe, Proceedings of the 43rd ACM SIGPLAN International Conference on Programming Language Design and Implementation 269 (2022) ISBN:9781450392655.
[15] Tomislav Begušić, Johnnie Gray, and Garnet Kin-Lic Chan, "Fast and converged classical simulations of evidence for the utility of quantum computing before fault tolerance", Science Advances 10 3, eadk4321 (2024).
[16] Thien Nguyen, Dmitry Lyakh, Eugene Dumitrescu, David Clark, Jeff Larkin, and Alexander McCaskey, "Tensor Network Quantum Virtual Machine for Simulating Quantum Circuits at Exascale", ACM Transactions on Quantum Computing 4 1, 1 (2023).
[17] Alistair W R Smith, A J Paige, and M S Kim, "Faster variational quantum algorithms with quantum kernel-based surrogate models", Quantum Science and Technology 8 4, 045016 (2023).
[18] Jordi Tura, "Boosting simulation of quantum computers", Nature Computational Science 1 10, 638 (2021).
[19] Joseph Tindall and Matthew Fishman, "Gauging tensor networks with belief propagation", SciPost Physics 15 6, 222 (2023).
[20] Hiryuki Ootomo, Hidetaka Manabe, Kenji Harada, and Rio Yokota, Lecture Notes in Computer Science 13948, 259 (2023) ISBN:978-3-031-32040-8.
[21] He-Liang Huang, Youwei Zhao, and Chu Guo, "How to Design a Classically Difficult Random Quantum Circuit for Quantum Computational Advantage Experiments", Intelligent Computing 3, 0079 (2024).
[22] Johnnie Gray and Garnet Kin-Lic Chan, "Hyperoptimized Approximate Contraction of Tensor Networks with Arbitrary Geometry", Physical Review X 14 1, 011009 (2024).
[23] Daiwei Zhu, Gregory D. Kahanamoku-Meyer, Laura Lewis, Crystal Noel, Or Katz, Bahaa Harraz, Qingfeng Wang, Andrew Risinger, Lei Feng, Debopriyo Biswas, Laird Egan, Alexandru Gheorghiu, Yunseong Nam, Thomas Vidick, Umesh Vazirani, Norman Y. Yao, Marko Cetina, and Christopher Monroe, "Interactive cryptographic proofs of quantumness using mid-circuit measurements", Nature Physics 19 11, 1725 (2023).
[24] Yong Liu, Yaojian Chen, Chu Guo, Jiawei Song, Xinmin Shi, Lin Gan, Wenzhao Wu, Wei Wu, Haohuan Fu, Xin Liu, Dexun Chen, Zhifeng Zhao, Guangwen Yang, and Jiangang Gao, "Verifying Quantum Advantage Experiments with Multiple Amplitude Tensor Network Contraction", Physical Review Letters 132 3, 030601 (2024).
[25] Andrea D’Urbano, Mario Angelelli, and Christian Catalano, Lecture Notes in Computer Science 14484, 60 (2024) ISBN:978-3-031-49268-6.
[26] Cameron Ibrahim, Danylo Lykov, Zichang He, Yuri Alexeev, and Ilya Safro, 2022 IEEE High Performance Extreme Computing Conference (HPEC) 1 (2022) ISBN:978-1-6654-9786-2.
[27] Sebastian Schlag, Tobias Heuer, Lars Gottesbüren, Yaroslav Akhremtsev, Christian Schulz, and Peter Sanders, "High-Quality Hypergraph Partitioning", ACM Journal of Experimental Algorithmics 27, 1 (2022).
[28] Sachin Kasture, Oleksandr Kyriienko, and Vincent E. Elfving, "Protocols for classically training quantum generative models on probability distributions", Physical Review A 108 4, 042406 (2023).
[29] Moritz Cygorek, Jonathan Keeling, Brendon W. Lovett, and Erik M. Gauger, "Sublinear Scaling in Non-Markovian Open Quantum Systems Simulations", Physical Review X 14 1, 011010 (2024).
[30] Robert Cimrman, "Fast evaluation of finite element weak forms using python tensor contraction packages", Advances in Engineering Software 159, 103033 (2021).
[31] Qingling Zhu, Sirui Cao, Fusheng Chen, Ming-Cheng Chen, Xiawei Chen, Tung-Hsun Chung, Hui Deng, Yajie Du, Daojin Fan, Ming Gong, Cheng Guo, Chu Guo, Shaojun Guo, Lianchen Han, Linyin Hong, He-Liang Huang, Yong-Heng Huo, Liping Li, Na Li, Shaowei Li, Yuan Li, Futian Liang, Chun Lin, Jin Lin, Haoran Qian, Dan Qiao, Hao Rong, Hong Su, Lihua Sun, Liangyuan Wang, Shiyu Wang, Dachao Wu, Yulin Wu, Yu Xu, Kai Yan, Weifeng Yang, Yang Yang, Yangsen Ye, Jianghan Yin, Chong Ying, Jiale Yu, Chen Zha, Cha Zhang, Haibin Zhang, Kaili Zhang, Yiming Zhang, Han Zhao, Youwei Zhao, Liang Zhou, Chao-Yang Lu, Cheng-Zhi Peng, Xiaobo Zhu, and Jian-Wei Pan, "Quantum computational advantage via 60-qubit 24-cycle random circuit sampling", Science Bulletin 67 3, 240 (2022).
[32] Yong (Alexander) Liu, Xin (Lucy) Liu, Fang (Nancy) Li, Haohuan Fu, Yuling Yang, Jiawei Song, Pengpeng Zhao, Zhen Wang, Dajia Peng, Huarong Chen, Chu Guo, Heliang Huang, Wenzhao Wu, and Dexun Chen, Proceedings of the International Conference for High Performance Computing, Networking, Storage and Analysis 1 (2021) ISBN:9781450384421.
[33] Feng Pan and Pan Zhang, "Simulation of Quantum Circuits Using the Big-Batch Tensor Network Method", Physical Review Letters 128 3, 030501 (2022).
[34] Justin Provazza, Klaas Gunst, Huanchen Zhai, Garnet K.-L. Chan, Toru Shiozaki, Nicholas C. Rubin, and Alec F. White, "Fast Emulation of Fermionic Circuits with Matrix Product States", Journal of Chemical Theory and Computation acs.jctc.4c00200 (2024).
[35] Danylo Lykov, Jonathan Wurtz, Cody Poole, Mark Saffman, Tom Noel, and Yuri Alexeev, "Sampling frequency thresholds for the quantum advantage of the quantum approximate optimization algorithm", npj Quantum Information 9 1, 73 (2023).
[36] Dorit Aharonov, Xun Gao, Zeph Landau, Yunchao Liu, and Umesh Vazirani, Proceedings of the 55th Annual ACM Symposium on Theory of Computing 945 (2023) ISBN:9781450399135.
[37] Jeffrey Marshall and Namit Anand, "Simulation of quantum optics by coherent state decomposition", Optica Quantum 1 2, 78 (2023).
[38] Yulin Wu, Wan-Su Bao, Sirui Cao, Fusheng Chen, Ming-Cheng Chen, Xiawei Chen, Tung-Hsun Chung, Hui Deng, Yajie Du, Daojin Fan, Ming Gong, Cheng Guo, Chu Guo, Shaojun Guo, Lianchen Han, Linyin Hong, He-Liang Huang, Yong-Heng Huo, Liping Li, Na Li, Shaowei Li, Yuan Li, Futian Liang, Chun Lin, Jin Lin, Haoran Qian, Dan Qiao, Hao Rong, Hong Su, Lihua Sun, Liangyuan Wang, Shiyu Wang, Dachao Wu, Yu Xu, Kai Yan, Weifeng Yang, Yang Yang, Yangsen Ye, Jianghan Yin, Chong Ying, Jiale Yu, Chen Zha, Cha Zhang, Haibin Zhang, Kaili Zhang, Yiming Zhang, Han Zhao, Youwei Zhao, Liang Zhou, Qingling Zhu, Chao-Yang Lu, Cheng-Zhi Peng, Xiaobo Zhu, and Jian-Wei Pan, "Strong Quantum Computational Advantage Using a Superconducting Quantum Processor", Physical Review Letters 127 18, 180501 (2021).
[39] Matthew Fishman, Steven White, and Edwin Stoudenmire, "The ITensor Software Library for Tensor Network Calculations", SciPost Physics Codebases 4 (2022).
[40] Lukas Burgholzer, Hartwig Bauer, and Robert Wille, 2021 IEEE International Conference on Quantum Computing and Engineering (QCE) 199 (2021) ISBN:978-1-6654-1691-7.
[41] Glen Evenbly, "A Practical Guide to the Numerical Implementation of Tensor Networks I: Contractions, Decompositions, and Gauge Freedom", Frontiers in Applied Mathematics and Statistics 8, 806549 (2022).
[42] He-Liang Huang, Xiao-Yue Xu, Chu Guo, Guojing Tian, Shi-Jie Wei, Xiaoming Sun, Wan-Su Bao, and Gui-Lu Long, "Near-term quantum computing techniques: Variational quantum algorithms, error mitigation, circuit compilation, benchmarking and classical simulation", Science China Physics, Mechanics & Astronomy 66 5, 250302 (2023).
[43] Xiao Mi, Pedram Roushan, Chris Quintana, Salvatore Mandrà, Jeffrey Marshall, Charles Neill, Frank Arute, Kunal Arya, Juan Atalaya, Ryan Babbush, Joseph C. Bardin, Rami Barends, Joao Basso, Andreas Bengtsson, Sergio Boixo, Alexandre Bourassa, Michael Broughton, Bob B. Buckley, David A. Buell, Brian Burkett, Nicholas Bushnell, Zijun Chen, Benjamin Chiaro, Roberto Collins, William Courtney, Sean Demura, Alan R. Derk, Andrew Dunsworth, Daniel Eppens, Catherine Erickson, Edward Farhi, Austin G. Fowler, Brooks Foxen, Craig Gidney, Marissa Giustina, Jonathan A. Gross, Matthew P. Harrigan, Sean D. Harrington, Jeremy Hilton, Alan Ho, Sabrina Hong, Trent Huang, William J. Huggins, L. B. Ioffe, Sergei V. Isakov, Evan Jeffrey, Zhang Jiang, Cody Jones, Dvir Kafri, Julian Kelly, Seon Kim, Alexei Kitaev, Paul V. Klimov, Alexander N. Korotkov, Fedor Kostritsa, David Landhuis, Pavel Laptev, Erik Lucero, Orion Martin, Jarrod R. McClean, Trevor McCourt, Matt McEwen, Anthony Megrant, Kevin C. Miao, Masoud Mohseni, Shirin Montazeri, Wojciech Mruczkiewicz, Josh Mutus, Ofer Naaman, Matthew Neeley, Michael Newman, Murphy Yuezhen Niu, Thomas E. O’Brien, Alex Opremcak, Eric Ostby, Balint Pato, Andre Petukhov, Nicholas Redd, Nicholas C. Rubin, Daniel Sank, Kevin J. Satzinger, Vladimir Shvarts, Doug Strain, Marco Szalay, Matthew D. Trevithick, Benjamin Villalonga, Theodore White, Z. Jamie Yao, Ping Yeh, Adam Zalcman, Hartmut Neven, Igor Aleiner, Kostyantyn Kechedzhi, Vadim Smelyanskiy, and Yu Chen, "Information scrambling in quantum circuits", Science 374 6574, 1479 (2021).
[44] Han-Sen Zhong, Yu-Hao Deng, Jian Qin, Hui Wang, Ming-Cheng Chen, Li-Chao Peng, Yi-Han Luo, Dian Wu, Si-Qiu Gong, Hao Su, Yi Hu, Peng Hu, Xiao-Yan Yang, Wei-Jun Zhang, Hao Li, Yuxuan Li, Xiao Jiang, Lin Gan, Guangwen Yang, Lixing You, Zhen Wang, Li Li, Nai-Le Liu, Jelmer J. Renema, Chao-Yang Lu, and Jian-Wei Pan, "Phase-Programmable Gaussian Boson Sampling Using Stimulated Squeezed Light", Physical Review Letters 127 18, 180502 (2021).
[45] Yaojian Chen, Yong Liu, Xinmin Shi, Jiawei Song, Xin Liu, Lin Gan, Chu Guo, Haohuan Fu, Jie Gao, Dexun Chen, and Guangwen Yang, Proceedings of the 28th ACM SIGPLAN Annual Symposium on Principles and Practice of Parallel Programming 148 (2023) ISBN:9798400700156.
[46] Akihiro Tabuchi, Satoshi Imamura, Masafumi Yamazaki, Takumi Honda, Akihiko Kasagi, Hiroshi Nakao, Naoto Fukumoto, and Kohta Nakashima, 2023 IEEE International Conference on Quantum Computing and Engineering (QCE) 959 (2023) ISBN:979-8-3503-4323-6.
[47] Jonathan Wurtz and Danylo Lykov, "Fixed-angle conjectures for the quantum approximate optimization algorithm on regular MaxCut graphs", Physical Review A 104 5, 052419 (2021).
[48] Sangchul Oh and Sabre Kais, "Comparison of quantum advantage experiments using random circuit sampling", Physical Review A 107 2, 022610 (2023).
[49] Dominik Hangleiter and Jens Eisert, "Computational advantage of quantum random sampling", Reviews of Modern Physics 95 3, 035001 (2023).
[50] Reza Haghshenas, Johnnie Gray, Andrew C. Potter, and Garnet Kin-Lic Chan, "Variational Power of Quantum Circuit Tensor Networks", Physical Review X 12 1, 011047 (2022).
[51] Ruojing Peng, Johnnie Gray, and Garnet Kin-Lic Chan, "Arithmetic circuit tensor networks, multivariable function representation, and high-dimensional integration", Physical Review Research 5 1, 013156 (2023).
[52] Martin Roa-Villescas and Jin-Guo Liu, "TensorInference: A Julia package for tensor-based probabilistic inference", Journal of Open Source Software 8 90, 5700 (2023).
[53] Reza Haghshenas, "Optimization schemes for unitary tensor-network circuit", Physical Review Research 3 2, 023148 (2021).
[54] Danylo Lykov, Ruslan Shaydulin, Yue Sun, Yuri Alexeev, and Marco Pistoia, Proceedings of the SC '23 Workshops of The International Conference on High Performance Computing, Network, Storage, and Analysis 1443 (2023) ISBN:9798400707858.
[55] Sergio Sanchez-Ramirez, Javier Conejero, Francesc Lordan, Anna Queralt, Toni Cortes, Rosa M Badia, and Artur Garcia-Saez, 2021 IEEE/ACM Second International Workshop on Quantum Computing Software (QCS) 1 (2021) ISBN:978-1-7281-8674-0.
[56] Ümit Çatalyürek, Karen Devine, Marcelo Faraj, Lars Gottesbüren, Tobias Heuer, Henning Meyerhenke, Peter Sanders, Sebastian Schlag, Christian Schulz, Daniel Seemaier, and Dorothea Wagner, "More Recent Advances in (Hyper)Graph Partitioning", ACM Computing Surveys 55 12, 1 (2023).
[57] Alexander Zlokapa, Benjamin Villalonga, Sergio Boixo, and Daniel A. Lidar, "Boundaries of quantum supremacy via random circuit sampling", npj Quantum Information 9 1, 36 (2023).
[58] Jernej Rudi Finžgar, Aron Kerschbaumer, Martin J.A. Schuetz, Christian B. Mendl, and Helmut G. Katzgraber, "Quantum-Informed Recursive Optimization Algorithms", PRX Quantum 5 2, 020327 (2024).
[59] Shi-Xin Zhang, Jonathan Allcock, Zhou-Quan Wan, Shuo Liu, Jiace Sun, Hao Yu, Xing-Han Yang, Jiezhong Qiu, Zhaofeng Ye, Yu-Qin Chen, Chee-Kong Lee, Yi-Cong Zheng, Shao-Kai Jian, Hong Yao, Chang-Yu Hsieh, and Shengyu Zhang, "TensorCircuit: a Quantum Software Framework for the NISQ Era", Quantum 7, 912 (2023).
[60] Ryan L. Mann, "Simulating quantum computations with Tutte polynomials", npj Quantum Information 7 1, 141 (2021).
[61] Yijia Wang, Yuwen Ebony Zhang, Feng Pan, and Pan Zhang, "Tensor Network Message Passing", Physical Review Letters 132 11, 117401 (2024).
[62] Angus Lowe, Matija Medvidović, Anthony Hayes, Lee J. O'Riordan, Thomas R. Bromley, Juan Miguel Arrazola, and Nathan Killoran, "Fast quantum circuit cutting with randomized measurements", Quantum 7, 934 (2023).
[63] Dian-Teng Chen, Phillip Helms, Ashlyn R. Hale, Minseong Lee, Chenghan Li, Johnnie Gray, George Christou, Vivien S. Zapf, Garnet Kin-Lic Chan, and Hai-Ping Cheng, "Using Hyperoptimized Tensor Networks and First-Principles Electronic Structure to Simulate the Experimental Properties of the Giant {Mn84} Torus", The Journal of Physical Chemistry Letters 13 10, 2365 (2022).
[64] Meng Zhang, Chao Wang, Shaojun Dong, Hao Zhang, Yongjian Han, and Lixin He, "Entanglement entropy scaling of noisy random quantum circuits in two dimensions", Physical Review A 106 5, 052430 (2022).
[65] Shaowen Li, Yusuke Kimura, Hiroyuki Sato, and Masahiro Fujita, "Parallelizing Quantum Simulation With Decision Diagrams", IEEE Transactions on Quantum Engineering 5, 1 (2024).
[66] Wei Xu, Weiqin Tong, and Xiaoyang Liu, 2024 4th International Conference on Neural Networks, Information and Communication (NNICE) 133 (2024) ISBN:979-8-3503-9437-5.
[67] Ying Li, Ze-Yao Han, Chao-Jian Li, Jin Lü, Xiao Yuan, and Bu-Jiao Wu, "Review on quantum advantages of sampling problems", Acta Physica Sinica 70 21, 210201 (2021).
[68] Aaron Sander, Lukas Burgholzer, and Robert Wille, 2023 IEEE International Conference on Quantum Computing and Engineering (QCE) 283 (2023) ISBN:979-8-3503-4323-6.
[69] K. Kechedzhi, S.V. Isakov, S. Mandrà, B. Villalonga, X. Mi, S. Boixo, and V. Smelyanskiy, "Effective quantum volume, fidelity and computational cost of noisy quantum processing experiments", Future Generation Computer Systems 153, 431 (2024).
[70] Dmitry I. Lyakh, Thien Nguyen, Daniel Claudino, Eugene Dumitrescu, and Alexander J. McCaskey, "ExaTN: Scalable GPU-Accelerated High-Performance Processing of General Tensor Networks at Exascale", Frontiers in Applied Mathematics and Statistics 8, 838601 (2022).
[71] Xin Hong, Xiangzhen Zhou, Sanjiang Li, Yuan Feng, and Mingsheng Ying, "A Tensor Network based Decision Diagram for Representation of Quantum Circuits", ACM Transactions on Design Automation of Electronic Systems 27 6, 1 (2022).
[72] Cupjin Huang, Fang Zhang, Michael Newman, Xiaotong Ni, Dawei Ding, Junjie Cai, Xun Gao, Tenghui Wang, Feng Wu, Gengyan Zhang, Hsiang-Sheng Ku, Zhengxiong Tian, Junyin Wu, Haihong Xu, Huanjun Yu, Bo Yuan, Mario Szegedy, Yaoyun Shi, Hui-Hai Zhao, Chunqing Deng, and Jianxin Chen, "Efficient parallelization of tensor network contraction for simulating quantum computation", Nature Computational Science 1 9, 578 (2021).
[73] Hao-Kai Zhang, Shuo Liu, and Shi-Xin Zhang, "Absence of Barren Plateaus in Finite Local-Depth Circuits with Long-Range Entanglement", Physical Review Letters 132 15, 150603 (2024).
[74] Mari Carmen Bañuls, "Tensor Network Algorithms: A Route Map", Annual Review of Condensed Matter Physics 14 1, 173 (2023).
[75] Lars S. Madsen, Fabian Laudenbach, Mohsen Falamarzi. Askarani, Fabien Rortais, Trevor Vincent, Jacob F. F. Bulmer, Filippo M. Miatto, Leonhard Neuhaus, Lukas G. Helt, Matthew J. Collins, Adriana E. Lita, Thomas Gerrits, Sae Woo Nam, Varun D. Vaidya, Matteo Menotti, Ish Dhand, Zachary Vernon, Nicolás Quesada, and Jonathan Lavoie, "Quantum computational advantage with a programmable photonic processor", Nature 606 7912, 75 (2022).
[76] Manuel S Rudolph, Jing Chen, Jacob Miller, Atithi Acharya, and Alejandro Perdomo-Ortiz, "Decomposition of matrix product states into shallow quantum circuits", Quantum Science and Technology 9 1, 015012 (2024).
[77] Lars Gottesbüren, Tobias Heuer, Nikolai Maas, Peter Sanders, and Sebastian Schlag, "Scalable High-Quality Hypergraph Partitioning", ACM Transactions on Algorithms 20 1, 1 (2024).
[78] Oliver Lunt, Jonas Richter, and Arijeet Pal, Quantum Science and Technology 251 (2022) ISBN:978-3-031-03997-3.
[79] Thomas Ayral, Thibaud Louvet, Yiqing Zhou, Cyprien Lambert, E. Miles Stoudenmire, and Xavier Waintal, "Density-Matrix Renormalization Group Algorithm for Simulating Quantum Circuits with a Finite Fidelity", PRX Quantum 4 2, 020304 (2023).
[80] Nicholas C. Rubin, Klaas Gunst, Alec White, Leon Freitag, Kyle Throssell, Garnet Kin-Lic Chan, Ryan Babbush, and Toru Shiozaki, "The Fermionic Quantum Emulator", Quantum 5, 568 (2021).
[81] Nicholas H. Stair and Francesco A. Evangelista, "QForte: An Efficient State-Vector Emulator and Quantum Algorithms Library for Molecular Electronic Structure", Journal of Chemical Theory and Computation 18 3, 1555 (2022).
[82] M. R. Perelshtein, A. I. Pakhomchik, Ar. A. Melnikov, M. Podobrii, A. Termanova, I. Kreidich, B. Nuriev, S. Iudin, C. W. Mansell, and V. M. Vinokur, "NISQ-compatible approximate quantum algorithm for unconstrained and constrained discrete optimization", Quantum 7, 1186 (2023).
[83] Abhinav Deshpande, Arthur Mehta, Trevor Vincent, Nicolás Quesada, Marcel Hinsche, Marios Ioannou, Lars Madsen, Jonathan Lavoie, Haoyu Qi, Jens Eisert, Dominik Hangleiter, Bill Fefferman, and Ish Dhand, "Quantum computational advantage via high-dimensional Gaussian boson sampling", Science Advances 8 1, eabi7894 (2022).
[84] Lukas Burgholzer, Alexander Ploier, and Robert Wille, 2022 Design, Automation & Test in Europe Conference & Exhibition (DATE) 64 (2022) ISBN:978-3-9819263-6-1.
[85] Fang Li, Xin Liu, Yong Liu, Pengpeng Zhao, Yuling Yang, Honghui Shang, Weizhe Sun, Zhen Wang, Enming Dong, and Dexun Chen, Proceedings of the International Conference for High Performance Computing, Networking, Storage and Analysis 1 (2021) ISBN:9781450384421.
[86] Meng Zhang, Chao Wang, and Yongjian Han, "Noisy Random Quantum Circuit Sampling and its Classical Simulation", Advanced Quantum Technologies 6 7, 2300030 (2023).
[87] Takato Mori, Hidetaka Manabe, and Hiroaki Matsueda, "Entanglement distillation toward minimal bond cut surface in tensor networks", Physical Review D 106 8, 086008 (2022).
[88] Trevor Vincent, Lee J. O'Riordan, Mikhail Andrenkov, Jack Brown, Nathan Killoran, Haoyu Qi, and Ish Dhand, "Jet: Fast quantum circuit simulations with parallel task-based tensor-network contraction", Quantum 6, 709 (2022).
[89] Alexander M. Dalzell, Sam McArdle, Mario Berta, Przemyslaw Bienias, Chi-Fang Chen, András Gilyén, Connor T. Hann, Michael J. Kastoryano, Emil T. Khabiboulline, Aleksander Kubica, Grant Salton, Samson Wang, and Fernando G. S. L. Brandão, "Quantum algorithms: A survey of applications and end-to-end complexities", arXiv:2310.03011, (2023).
[90] Cupjin Huang, Fang Zhang, Michael Newman, Junjie Cai, Xun Gao, Zhengxiong Tian, Junyin Wu, Haihong Xu, Huanjun Yu, Bo Yuan, Mario Szegedy, Yaoyun Shi, and Jianxin Chen, "Classical Simulation of Quantum Supremacy Circuits", arXiv:2005.06787, (2020).
[91] Feng Pan and Pan Zhang, "Simulating the Sycamore quantum supremacy circuits", arXiv:2103.03074, (2021).
[92] Ramis Movassagh, "Quantum supremacy and random circuits", arXiv:1909.06210, (2019).
[93] Benjamin Villalonga, Murphy Yuezhen Niu, Li Li, Hartmut Neven, John C. Platt, Vadim N. Smelyanskiy, and Sergio Boixo, "Efficient approximation of experimental Gaussian boson sampling", arXiv:2109.11525, (2021).
[94] José Ramón Pareja Monturiol, David Pérez-García, and Alejandro Pozas-Kerstjens, "TensorKrowch: Smooth integration of tensor networks in machine learning", arXiv:2306.08595, (2023).
[95] Xun Gao, Marcin Kalinowski, Chi-Ning Chou, Mikhail D. Lukin, Boaz Barak, and Soonwon Choi, "Limitations of Linear Cross-Entropy as a Measure for Quantum Advantage", PRX Quantum 5 1, 010334 (2024).
[96] Alexander Zlokapa, Sergio Boixo, and Daniel Lidar, "Boundaries of quantum supremacy via random circuit sampling", arXiv:2005.02464, (2020).
[97] Feng Pan, Pengfei Zhou, Sujie Li, and Pan Zhang, "Contracting Arbitrary Tensor Networks: General Approximate Algorithm and Applications in Graphical Models and Quantum Circuit Simulations", Physical Review Letters 125 6, 060503 (2020).
[98] Xiaosi Xu, Simon Benjamin, Jinzhao Sun, Xiao Yuan, and Pan Zhang, "A Herculean task: Classical simulation of quantum computers", arXiv:2302.08880, (2023).
[99] Jin-Guo Liu, Lei Wang, and Pan Zhang, "Tropical Tensor Network for Ground States of Spin Glasses", Physical Review Letters 126 9, 090506 (2021).
[100] Alex Townsend-Teague and Konstantinos Meichanetzidis, "Simplification Strategies for the Qutrit ZX-Calculus", arXiv:2103.06914, (2021).
[101] Danylo Lykov, Ruslan Shaydulin, Yue Sun, Yuri Alexeev, and Marco Pistoia, "Fast Simulation of High-Depth QAOA Circuits", arXiv:2309.04841, (2023).
[102] Xin Hong, Xiangzhen Zhou, Sanjiang Li, Yuan Feng, and Mingsheng Ying, "A Tensor Network based Decision Diagram for Representation of Quantum Circuits", arXiv:2009.02618, (2020).
[103] Harun Bayraktar, Ali Charara, David Clark, Saul Cohen, Timothy Costa, Yao-Lung L. Fang, Yang Gao, Jack Guan, John Gunnels, Azzam Haidar, Andreas Hehn, Markus Hohnerbach, Matthew Jones, Tom Lubowe, Dmitry Lyakh, Shinya Morino, Paul Springer, Sam Stanwyck, Igor Terentyev, Satya Varadhan, Jonathan Wong, and Takuma Yamaguchi, "cuQuantum SDK: A High-Performance Library for Accelerating Quantum Science", arXiv:2308.01999, (2023).
[104] John Brennan, Momme Allalen, David Brayford, Kenneth Hanley, Luigi Iapichino, Lee J. O'Riordan, Myles Doyle, and Niall Moran, "Tensor Network Circuit Simulation at Exascale", arXiv:2110.09894, (2021).
[105] Feng Pan, Hanfeng Gu, Lvlin Kuang, Bing Liu, and Pan Zhang, "Efficient Quantum Circuit Simulation by Tensor Network Methods on Modern GPUs", arXiv:2310.03978, (2023).
[106] Roman Schutski, Taras Khakhulin, Ivan Oseledets, and Dmitry Kolmakov, "Simple heuristics for efficient parallel tensor contraction and quantum circuit simulation", Physical Review A 102 6, 062614 (2020).
[107] Hiroyuki Ootomo, Hidetaka Manabe, Kenji Harada, and Rio Yokota, "Quantum Circuit Simulation by SGEMM Emulation on Tensor Cores and Automatic Precision Selection", arXiv:2303.08989, (2023).
[108] Zhimin Wang, Zhaoyun Chen, Shengbin Wang, Wendong Li, Yongjian Gu, Guoping Guo, and Zhiqiang Wei, "A quantum circuit simulator and its applications on Sunway TaihuLight supercomputer", arXiv:2008.07140, (2020).
[109] Willow Ahrens, Fredrik Kjolstad, and Saman Amarasinghe, "An Asymptotic Cost Model for Autoscheduling Sparse Tensor Programs", arXiv:2111.14947, (2021).
[110] Lars Gottesbüren, Tobias Heuer, Nikolai Maas, Peter Sanders, and Sebastian Schlag, "Scalable High-Quality Hypergraph Partitioning", arXiv:2303.17679, (2023).
[111] Jeffrey M. Dudek and Moshe Y. Vardi, "Parallel Weighted Model Counting with Tensor Networks", arXiv:2006.15512, (2020).
[112] Tuomas Laakkonen, Konstantinos Meichanetzidis, and John van de Wetering, "Picturing Counting Reductions with the ZH-Calculus", arXiv:2304.02524, (2023).
[113] Linjian Ma and Chao Yang, "Low Rank Approximation in Simulations of Quantum Algorithms", arXiv:2104.11396, (2021).
[114] Tobias Heuer, "A Direct k-Way Hypergraph Partitioning Algorithm for Optimizing the Steiner Tree Metric", arXiv:2309.16694, (2023).
[115] Elisabeth Wybo and Martin Leib, "Vanishing performance of the parity-encoded quantum approximate optimization algorithm applied to spin-glass models", arXiv:2311.02151, (2023).
[116] Chi-Ning Chou, "The Computational Lens: from Quantum Physics to Neuroscience", arXiv:2310.20539, (2023).
[117] Shaan Nagy, Roger Paredes, Jeffrey M. Dudek, Leonardo Dueñas-Osorio, and Moshe Y. Vardi, "Ising model partition-function computation as a weighted counting problem", Physical Review E 109 5, 055301 (2024).
The above citations are from Crossref's cited-by service (last updated successfully 2024-05-12 08:45:03) and SAO/NASA ADS (last updated successfully 2024-05-12 08:45:04). The list may be incomplete as not all publishers provide suitable and complete citation data.
This Paper is published in Quantum under the Creative Commons Attribution 4.0 International (CC BY 4.0) license. Copyright remains with the original copyright holders such as the authors or their institutions.